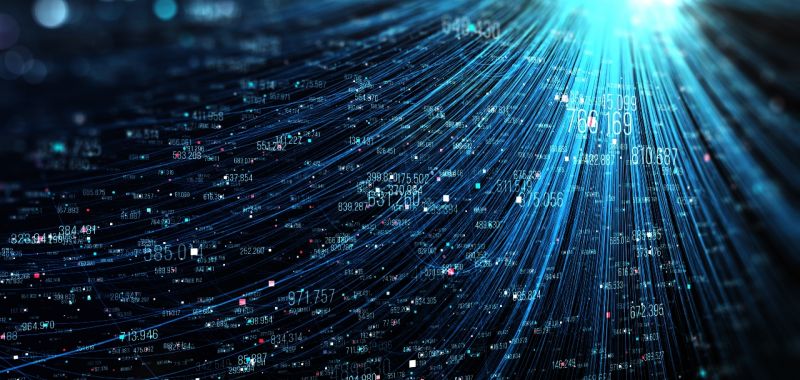
Materials science unleashed: ROSA renders supercomputers redundant
Machine learning informed by a new set of digital attributes will help materials scientists to discover key information without a supercomputer, boosting research ranging from solar energy to green hydrogen.
Generating important data about how materials are expected to behave has previously required complicated calculations such as density functional theory (DFT), which can take hours or even days using a supercomputer. Such in-demand infrastructure is rare and hard to access, limiting our capacity to uncover new knowledge and solve global problems.
Machine learning models offer a quicker, cheaper alternative to supercomputing. By using a small, incomplete part of a full DFT calculation, they can be trained to rapidly generate the same accurate results – and save time, money, and energy in the process.
Large, raw data sets about materials are now available for analysis by machine learning models, but first it’s necessary to pick a group of attributes or ‘descriptors’ that can map the structure of a material against its other relevant properties.
That’s where ROSA (robust one-shot ab initio) descriptors come in.
Generated using the energetic qualities of a material, researchers from the ARC Centre of Excellence in Exciton Science have used ROSA descriptors to train machine learning models with impressive results.
Their work has been published in the Journal of Cheminformatics and is available here.
First author Dr Sherif Abdulkader Tawfik Abbas, a member of Deakin University’s Institute for Frontier Materials, said: “We thought, how about instead of the whole DFT calculation, we just do one little step of it?’
“We give it just a flavour and then we rely on the machine learning model to fill in the gap. From the flavour to the full meal.”
Developed in conjunction with Professor Salvy Russo of RMIT University, the descriptors have produced accurate predictions about the characteristics of crystals, metal-organic frameworks and molecules.
“The cool thing about this work is how many properties these descriptors were able to predict,” Sherif said.
ROSA descriptors have been able to predict an abundance of useful data with a high degree of accuracy, including band gap and formation energies, as well as mechanical and vibrational properties of materials, and even molecular properties.
“Formation energy is necessary for predicting the thermodynamic stability of a material,” Sherif said.
“The mechanical property tells us how hard the material is in response to mechanical strain and the vibrational property tells us how stable a material is in response to small thermal disturbances.”
The information about molecules generated via ROSA is particularly helpful, with the models predicting the HOMO-LUMO gap and the free energy of molecules, which are equivalent to the band gap and formation energy respectively in materials. The isotropic polarizability, which is an important property of molecules, was also predicted - all with a very high degree of accuracy.
According to Sherif, ROSA should prove versatile and effective for a wide range of chemists and materials scientists, including researchers working on solar energy, catalysis for efficient chemical reactions and semiconductor devices.
He said: “What can people use ROSA for? Anyone who is applying machine learning to material science. There is a large range of descriptors available in the literature. People use them for all sorts of materials properties prediction. Anyone who’s dealing with materials or molecules can definitely make use of ROSA.”